Training of Trainers Workshop on Climate Change Science – Summary of Proceedings
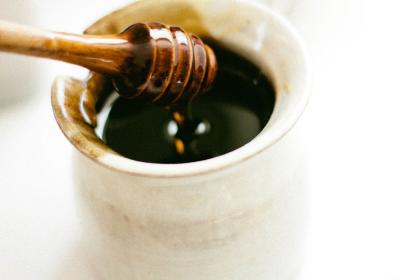
- Level: Introductory
- Time commitment: 1-2 hours
- Learning product: Report on a workshop
- Sector: Multi-sector
- Language: English
- Certificate available: No
Session 1: Setting the Stage and Exploration
This is a workshop report from Training of Trainers workshop on Climate Analysis held in April 2008 to support the technical assistance teams to the ACCCA project which is coordinated by UNITAR.
Monday 31st March
The workshop began with some introductions and a session on what each participant expected to learn from the 2 weeks. Participants discussed their aims and expectations for the material to be covered and the outputs from the 2 weeks. Several main categories were identified (these are also depicted in Figure 1 below):
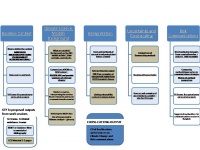
Risk Communication:
- Learn from others’ experience of communicating risk
- Come up with innovative risk communication strategies
- Put together guidance on risk communication as a process, which can used when working with the project teams.
Climate Uncertainty
- A better understanding of the uncertainties in climate prediction and the downscaling technique.
- Guidance document comparing downscaling techniques: RCMs vs Empirical downscaling.
- Collection of key resources on downscaling and climate science, both CSAG publications and others.
Climate Interpretation
- Develop guidance and questions to support the technical backstopping teams working with ACCCA teams.
- Guidance for projects on how to interpret the output of the CCE tool
- Learn how to interpret climate data so that it is relevant and applicable when communicated at the community level.
- Exploring the coupling of socio-economic and climate data to understand risks. How to go about understanding the context in which the climate data are to be used.
Risk Communication and Climate Change
The group then began to discuss some of the issues around risk communication, and identified several areas within risk communication on which to focus over the 2 weeks:
- Assessment vs. positive communication
- What are projects’ interpretations of their communication strategy?
- Check in project documents
- Are teams’ communication strategies followed through in their proposals/ project documents?
- How to increase communication between ACCCA teams on successful risk communication strategies
Session 2: Risk Communications
Initial Outputs Defined
A number of outputs were also suggested, some as short-term outputs relating to the 2 weeks, and some to be worked on over the next few months. These were:
1. Selecting methods of dissemination
Lead: Moliehi Shale
- Compile table of the different (applicable) methods and where they are useful and have been used – build on Annie’s table.
- Supply communication methods e.g how to write a policy brief
- Key issues/questions to have in mind (as technical team) when speaking to ACCCA teams. Provide checklist or examples of some of the issues that might be useful when trying to get feedback from the country teams.
2. Understanding the risk and consequences for the area
Lead: Fernanda Zermoglio
- Put together examples of risks and consequences for the area – these are very important when speaking to people
- Write list of questions for the technical assistants to work through with their project teams. Put together a 2 page guidance document on how to support teams with risk communication.
- This could include developing the communication material and testing so that the material is targeted and relevant.
3. Identifying the purpose of communication; who is the target audience?
Lead: Moliehi Shale and Gina Ziervogel
- Document on who the target is, what information they may want and how to link the different stakeholders (document to later be translated into French).
- What will make sense or be relevant to a particular audience (targeted communication)?
- How would you communicate AR4 Africa summary to different audiences e.g policy makers, farmers etc.?
- Write a generic brief on positive communication methods – associated challenges, outlining contexts where certain methods have been successful. Assessment vs. positive communication – what are the pathways to generate targeted messages and what is the most suitable delivery or dissemination method? (based on the table)
4. Two-page brief on the process of risk communication
Lead: Boubacar Fall and Moliehi Shale
5. Increasing communication between ACCCA teams:
- Participate in training workshops – Cape Town (April)
- Do field visits – Gina Ziervogel in Malawi (April); Fernanda (with Ben Smith) in Mali (April)
- Linking to vulnerability – We Adapt and Vulnerability Net. Use wikiADAPT as a place to share information and experiences.
- Selection of methods and tools for development of communication material and dialogues
- Create and share guidance material and documents
- Develop an annotated bibliography of risk communication material; outlining its strengths and weaknesses [Boubacar Fall and Moliehi Shale]
- Pull in existing information and prescribe criteria for selection of method(s)
It was also noted, however that the main focus of the 2 weeks was to be climate analysis rather than risk communication.
Session 3: Exploring the Context
In the afternoon Fernanda talked to the group about the importance of developing and understanding the baseline vulnerability for a specific context before looking at future climate changes. By understanding the context of an area, and the factors which cause vulnerability in the present, it is then possible to begin to identify which of these factors are sensitive to changes in climate, and how projected climate changes might impact on these variables. A good way to understand this context is to ask a series of questions about the region. In the ACCCA context this means that technical support to projects should have a series of questions that they can ask the project teams in order to increase their the understanding of the teams about the context of the project.
Tuesday 1st April
On Tuesday morning Belynda Petrie from the OneWorld group in Cape Town came to talk to the participants about their work on a 4-5 year adaptation programme with the UK Department for International Development (DFID). Initial work on a project in Tanzania was presented and was a useful way of grounding in case study some of the previous day’s work on the importance of understanding the baseline vulnerability of a region.
Belynda emphasised the view that climate change acts as one of many stressors and does not act in isolation from other factors which also create vulnerability. This role as a stress multiplier can make it hard to distinguish between climate impacts and the impact of other stressors. She explained some of the context for Tanzania, including issues of dependence on agriculture, small civil society, conflict bewteen different users over water resources and regional conflict causing an influx of migrants into Tanzania. Water was identified as the major resource issue. The OneWorld team identified 4 different levels of climate change impacts:
- Direct physical climate effects, for example precipitation changes.
- Secondary effects on variables such as evapotranspiration and soil moisture.
- Tertiary effects on crops and cropping cycles due to the changes in soil moisture.
- Fourth level effects of the changes in crop yields and cycles on human health and livelihoods.
This highlights that there is a complex chain of impacts between initial physical changes in climate and eventual impacts on human societies. Belynda also stressed the importance of involving local communities and stakeholders in vulnerability analyses, as these are the people who have the best knowledge of their socio-economic context. This links to the importance of building on indigenous knowledge in adaptation strategies. People’s perception of climate change is also important to understand, as this influences the way that they adopt different adaptation strategies. If you can understand the factors that influence perception it is possible to target communications so that are more successful. As trust is an important factor in the communication process, in many cases it will be most appropriate to work through organisations with a history of working with the community.
After lunch the participants spent some time individually working on developing the context for different ACCCA projects. This was achieved through targeted internet searches of websites compiled by Fernanda, and use of the AWhere software and ACCCA project documents. Shuyu Wang worked on Mongolia project, Moussa Na Abou contextualized the Tanzania pilot project, Ben Smith focused on the Bangladesh project, Boubacar Fall worked on Cameroon project and Moliehi Shale analyzed the Malawi project baseline. Participants then presented briefly the initial factors that they had found to be important for their project. For the findings from these baseline analyses, which continued throughout the 2 weeks, please see the section on presentations from Thursday 10th.
Session 5: Introduction to Climate Change
Wednesday 2nd April
The day’s sessions were focussed on building understanding of the large-scale processes and feedbacks in the climate system before looking at climate change and model predictions specifically on Thursday. The day was split into 3 main sessions; a presentation from Peter Johnston covering basic climatological principles, a presentation from Chris Lennard on the processes driving ENSO and the monsoon system, and then a series of presentations from CSAG students on different feedbacks in the climate system.
Basic Climatology
Peter Johnston of CSAG provided a useful introduction to the basic concepts in climatology. The concepts of radiative forcing and the radiation budget of the earth were introduced, along with ideas of heating and convection in order to set the background and begin explaining broad scale patterns of circulation. Peter explained how heating air at the equator forces it to rise and spread towards the pole, before it cools and begins to descend around 30ï‚°N/S creating a band of high pressure with limited precipitation that causes the bands of deserts found around these latitudes. Pressure differences were introduced as driving winds and broad scale patterns of circulation, as well as the Coriolis effect in order to explain observed patterns of circulation and the circular motion found therein. The inter-tropical convergence zone (ITCZ), which is the key driver of precipitation across a large area of the tropics was examined, and the reasons for its seasonal migration and inter-annual variation explored. The participants were very interested in the ways in which variability in the position of the ITCZ drives rainfall variability in many locations over Africa in particular, and there was a good discussion around how climate change might influence the position of the ITCZ, and potentially cause the expansion of certain arid zones.
Other issues such as the way land-use changes can affect climate at a more local scale, the changes in climate caused by topography and natural climate variability were also touched upon. Climate variability was of strong interest to the participants, and it was noted that a lot of the work in the ACCCA projects is actually aimed at increasing capacity to adapt to this variability, but with a focus on how climate change will affect the system.
ENSO and Monsoon processes and seasonal forecasting.
Chris Lennard spoke to the participants about the monsoon system and the El Niño Southern Oscillation (ENSO), two of the most important processes in the climate system, with Mark Tadross answering questions where appropriate. The ACCCA projects in both Africa and Asia are strongly dependent on rains brought by the SE Asian and W. African monsoons respectively, so understanding the processes driving monsoon circulation is very important to start understanding the context of regional climate. In W Africa the warm, dry Harmattan wind, which is the opposite of monsoon circulation and occurs in winter, was also identified as a major issue in several projects, and the participants agreed the need for more information on this phenomenon. The AMMA project, which is examining all aspects of the W. African monsoon system, was briefly introduced, and identified as a source of data to be explored further.
Discussions of the variability of the monsoon and how the monsoon might change in the future were very interesting, and it was agreed that it is vital to understand and identify any trends in monsoon in order to successfully plan for adaptation. As part of this discussion, it was noted that the effect of El Niño on the monsoon is to weaken it and cause less rainfall, whereas the La Niña phase of the oscillation causes intense precipitation and flooding. It is these sorts of teleconnections in the climate system which must be understood in order to accurately understand the current nature of climate at the different project sites.
Chris continued by explaining the processes that cause the ENSO cycle, and some of the effects that this cycle has in different areas of the world. The 2-7 year cycle has large effects on other regions of the world, including W Africa and SE Asia. This was another example of why the ACCCA Technical Assistance teams need a good understanding of global climate processes in order to understand the context of the project teams. Variability in ENSO cycles was again discussed, as was how this might change in the future. This was a very fruitful discussion and examined in detail the accuracy of GCMs in modelling processes such as ENSO and its effects on global climate, and pointing out that if the models find this difficult to model for the present, it is hard to tell what changes in frequency and intensity there might be in the future. There was much discussion over the specifics of the vocabulary and processes used in climate models. An important distinction was made between forecasts, which are for the near-term and use current conditions such as sea surface temperatures (SSTs), to forecast climate up to a seasonal scale, and scenarios, which operate at a much longer timescale, show a range of possible future climates, and derive conditions dynamically by specifying only the concentration of GHGs in the atmosphere, and letting the model decide what conditions this will create. This led to discussions around the value and accuracy of seasonal forecasts, and also El Niño forecasts, with participants questioning what sort of variables are key for people’s livelihoods – for example onset of the rainy season rather than average precipitation. The use of probabilities in forecasting seasonal rainfall was examined, in particular in the context of accuracy and use for livelihood strategies. More extreme events were also raised as an issue, and Mark Tadross and Chris explained why events such as hurricanes and cyclones cannot be modelled by the GCMs as they are created at scales below the resolution at which the models operate.
There was a lot of interest in the way that climate models work, and their accuracy and limitations, for example the way in which models represent climate processes, and the reasons for this. These discussions set the background nicely for later talks with Mark Tadross and Bruce Hewitson about model uncertainty and inter-model comparisons.
CSAG honours students – Climate system feedbacks
We benefitted in the afternoon from several informative presentations from CSAG honours students on feedbacks in the climate system. Chris Lennard noted before starting that feedbacks influence the climate system, but do not drive it in the way that large-scale circulation does.
- Albedo – Albedo refers to the reflective properties of a material. Snow and ice can have an extremely high albedo, meaning they reflect the majority of radiation striking them back into space. In contrast, the albedo of ocean or bare earth is much lower, allowing it to absorb much more solar radiation. In practice this means that melting ice leads to a positive feedback effect because it exposes surfaces with a lower albedo, which capture solar energy more easily and warm, thus melting more ice. The opposite is also true, with increases in snow and ice reflecting more heat to space and cooling the system.
- Clouds –The effect of increased temperatures on cloud formation, and whether this will increase or decrease the amount of solar radiation reaching the surface is one of the greatest sources of uncertainty in GCMs and leads to different sensitivities depending on how it is modelled. Greater cloud cover will either warm the earth by acting as a blanket and keeping more heat in. or increased reflection of incoming radiation to space due to more cloud cover will cool the earth. Cloud processes operate below the spatial resolution of current GCMs, and also occur at short temporal scales, making them difficult to model. As a result, cloud formation in GCMs is prescribed from temperature and water vapour, and different models have different ways of doing this, which leads to uncertainty between them. Some RCMs are starting to resolve cloud processes dynamically, and the Earth Simulator model from Japan, operating at 10km resolution, should also start to do this.
- Water Vapour, Water vapour is the strongest greenhouse gas in the atmosphere, and its warming effect far outweighs that of methane and carbon dioxide. It is expected with confidence that a warmer atmosphere will hold more water vapour, and this will lead to still warmer temperatures. Water vapour can amplify other feedbacks – for example it will increase cloudiness, so strengthen any feedback effect from clouds.
- Lapse Rate, The lapse rate is the rate at which air cools as it rises in the atmosphere. The actual rate varies depending on atmospheric conditions such as moisture content. This is another factor which is prescribed in GCMs rather than modelled dynamically. The potential feedback arises because as air cools it emits less radiation back to space, therefore if the lapse rate were to increase so that air cooled faster, less radiation would be emitted to space and thus the earth would warm further.
- Carbon Dioxide release from Forests/Biomass – It is unsure what the effect of increasing temperatures will have on carbon storage in terrestrial biomass. Greater temperatures will increase respiration in plants, which will increase the production of carbon dioxide. However, increased temperatures also increase plant growth and photosynthesis, which decreases evapotranspiration and thus emission of carbon dioxide. The relative importance of these factors will determine whether the effect is a net increase or a net decrease in carbon dioxide from vegetation
- Permafrost – As peat permafrost melts it will become a greater sink for carbon dioxide because productivity of the ecosystem will increase. This will be outweighed by large emissions of methane as previously frozen plant matter decomposes, so the net effect will be a positive feedback as permafrost melts and emissions of methane increase.
- Wetlands – Wetlands are the world’s largest natural source of methane, and it’s largest natural sink of carbon dioxide. Whether or not wetlands are a net source or sink of greenhouse gasses depends on the type of plant, location of the wetland, rate of decomposition and many other factors. It is difficult to say what effect increased temperatures will have on the global balance of greenhouse gas emissions from wetlands.
- Methane hydrates/clathrates – Large amounts of methane are locked away in stable clathrate form at the bottom of continental shelves, due to low temperatures and high pressure. If bottom waters warm these clathrates could become destabilised and release large amounts of methane to the atmosphere, causing a large increase in temperatures.
- Carbon Dioxide fertilisation effect- It is difficult to model how the effects of increased levels on carbon dioxide will change overall uptake of carbon dioxide in plants. It is expected that initially plants will take up more carbon dioxide due to increased growth rates, but that under conditions of doubled CO2 they might start to emit more CO2 and actually become a net source of the gas.
- Ocean acidity – Carbon dioxide is absorbed into the ocean through a physical mechanism, as it moves from high concentrations in the atmosphere to lower concentrations in the ocean, and a biological pump, as plankton take up CO2 to grow, thus draw more into the ocean along an increased pressure gradient. As the ocean absorbs more carbon dioxide it becomes more acidic. This acts to reduce the draw-down of CO2 into the ocean and also means that more organisms with calcium carbonate shells are dissolved when they die before reaching the sea-bed, releasing more carbon dioxide to the atmosphere.
Feedbacks are complex and very difficult to model, and most are rarely included in GCMs, or are at best prescribed rather than modelled in a dynamic way.
Session 6: Climate Change Science and Modelling
Thursday 3rd April
Mark Tadross led the sessions on Thursday, which focussed on the detail of climate science and future projections. The sessions covered problems with climate data, an initial overview of the uncertainty in GCMs (before sessions from Bruce in week 2), the process of statistical downscaling, and a discussion of the differences between statistical and dynamical downscaling techniques. Much was also discussed about the way to use climate data and the need to identify the important climate variables relevant to the specific context being examined, which linked well to work earlier in the week on defining the baseline vulnerability before looking at climate projections.
Climate Change
Mark Tadross discuss the issues of climate change from a modelling perspective.
Global warming will not just be an increase in temperatures, but will affect some of the pressure systems that drive the climate system, for example the areas of high pressure around 30ï‚°N/S may intensify due to greater heating at the equator intensifying the downward limb of the Hadley cells. This could lead to a later onset of rains in these areas because the high pressure will take longer to break down.
The strengths and limitations of different data sources are very important to know, and the issue of missing or incomplete data was raised as an example. In many cases information about the data (metadata) is not available; this makes data comparisons difficult as the way the data were collected is not known. Some data points will be clearly suspect, for example minimum temperatures below 0C in tropical areas with no frosts, but the decision on what to leave out is largely a subjective one. 30 years data are needed in order to accurately look at trends, and 10 years daily data for a station is the minimum requirement to create the statistical relationships needed for downscaling.
Climate change will change the probability density function (frequency distribution) of temperatures and rainfall, and this has already been seen in many cases, for example night-time temperatures are becoming warmer.. Historical observations are no guarantee of future behaviour – the underlying processes may change. The near future is particularly difficult to predict because of the effect of various multi-decadal oscillations such as ENSO and the North Atlantic Oscillation (NAO). It is important to understand the reasons for observed trends, as they may in fact be due to non-climatic factors such as changes in land-use.
Daily trends can be calculated by comparing observations for each day of the year over the last 50 years. This allows sub-seasonal trends to be identified, for example a shortening but intensification of the rainy season. This is particularly useful as it could then be compared to a seasonal calendar to look at the effects that the trend in the climate variable will have on agriculture. The historical context of changes in climate is vital in understanding future changes; long-term trends occurring in the present may in fact be more important for adaptation in many cases than projected change for 2050.
The baseline climate is vital for comparison of change with GCM projections; however climate is changing all the time, so there is an issue around what is an appropriate baseline. 1960-90 is often taken as the baseline climate, however in discussions it was noted that this may be of little use in communicating climate change to communities as this may not be what they see as their ‘normal’ climate.
GCM projections of climate change were introduced at this point for the first time, and Mark pointed out that it is important to look at when specific regions receive rainfall before interpreting IPCC results. For example Dec-Feb and Jun-Aug may not be the most important seasons, or a 10% decrease in dry season precipitation may in fact have little effect. The IPCC have tried to communicate areas where changes are more confident by indicating regions where more than 2/3 of models used agree on the sign of change expected (positive or negative). Areas of consistent change in precipitation represent the area of the ITCZ and strengthened rainfall; however there is much greater uncertainty over changes in the transition areas where the ITCZ may change its movement. Unfortunately these are also the semi-arid areas which are most vulnerable to changes in rainfall.
Much of the difference in output between GCMs is due to the way that they parameterize different variable. This means that for certain variables, such as precipitation, the models can’t use their internal physics to make rainfall, so they define a relationship between, for example, humidity in the atmosphere and rainfall. Different models use different relationships, so their output varies. Different internal structures in GCMs also means that they position boundaries between wetting and drying in different areas, and this also accounts for much of the variation. Mark explained that it is very difficult to choose between models, or pick a ‘best’ model. The work done at CSAG shows that the skill of a GCM in simulating current climate cannot be taken as indicator of how good it is, as the change seen in the models under anthropogenic forcing does not depend on starting conditions. Models with different simulations of current climate which capture the broad processes show similar changes into the future, and it is this change that is taken and applied to current observed conditions.
It is important to know that GCM skill is at the broader scale; they do not perform well at the level of an individual grid cell. These grid cells are also averages of a large area (often 300km2) which can be very heterogeneous in topography and climate so they must not be interpreted as if they represent a point. GCMs must at least capture the 1st order responses, such as the position of the ITCZ and major pressure systems, of the atmosphere in order to be credible, it is at the level of 2cnd order responses such as changes in position or frequency, which differences occur. One of the major areas for improvement in GCMs is the way they model oscillations in the climate system such as ENSO and the NAO, which can have large effects on regional climate.
Downscaling
Mark Tadross
In order to overcome the difficulties of using GCMs to look at climate change at a sub-regional level, 2 downscaling techniques have been developed; Dynamical downscaling and Empirical downscaling.
Dynamical downscaling refers to regional climate models (RCMs). These models are run within GCMs (‘nested’ in GCMs) and take the GCM conditions as the conditions at their edges but run their own physical processes in between. RCMs typically have a resolution of 25-50km and are designed to capture local processes and feedbacks, which is a major strength. They still parameterize certain variables such as rainfall, however, and are computationally very heavy to run; even the easiest RCMs to run take 2 months to generate a control and future climate for a region based on initial conditions from 1 GCM. This means that it is very difficult to downscale RCMs for multiple GCMs and to compare how different RCMs perform. Using one RCM in one GCM only gives you one possible future, rather than the larger range of possibilities. There are large differences between results from different RCMs so it is important not just to rely on the results of 1 RCM run in 1 GCM. For ACCCA purposes, unless RCM data is available for the region as a comparison to empirically downscaled methods, then it is impractical to use them.
Empirical downscaling is based on the assumption that large-scale (synoptic) circulation is the main driver of local climatic conditions, and you can derive relationships between this synoptic circulation and local conditions. Empirical downscaling takes the aspect of climate which GCMs simulate most accurately -the synoptic circulation – and translates this into local results. Empirical downscaling captures the 1st order response of the climate system but doesn’t incorporate feedbacks. For most sites, however, the response from synoptic forcing will be much more important than the feedbacks in the system. The local variability which influences local climate can be accounted for by introducing a semi-random (stochastic) element into the procedure. The strength of empirical downscaling is that it does not require much computing power as once the data have been downscaled they can be disseminated, it allows easy comparison of many different GCM projections and it provides data at the level of individual stations This means that the range of uncertainty in the projections can be compared to look for where the models agree on the sign of change, thus more confident decisions can be made. It is important to relate the results of the downscaling back to larger scale processes; is there a physical process which could plausibly explain the changes seen in the downscaling? It is worth noting that the 2 main methods for empirical downscaling, those developed by Bruce Hewitson and Rob Wilby, are consistent in their results.
Mark noted the importance of looking at the evolution of changes over time, and not just the average of a time slice, in interpreting changes. There may be different physical mechanisms responsible for a projected decrease in early season rainfall and increase in late season rainfall which cause the changes to happen at different times. In this example looking at 2050 might show an early season decrease and late season increase, but the rainy season staying the same length, whereas actually early season decrease occurs earlier than the late season increase, so there is a period with a reduced rainy season prior to 2050.
Linking back to the work on setting the context of vulnerability from the first day of the workshop, Mark described the steps to go through in a climate change analysis, with a case study of work over southern Africa. The initial questions to answer before starting to an analysis of climate change were:
- What aspects of climate are you vulnerable to?
- What data do you have to characterise current hazards?
- To what extent can you characterise future climate?
- Which crop varieties are most important?
- Do you need to use climate data to do impact studies?
- What are the relevant agricultural details? For example which crops are most important? What is the phenology of the crop? Is it rain fed or irrigated? Are there agricultural statistics available?
The answers to these questions will influence the type of climate data (daily, monthly, seasonal) needed and the appropriate climate variables to look at. The overarching message is that vulnerability is context specific and that the climate analysis must be based on a sound understanding of the local context.
Southern Africa example
Mark described some examples from his work in Southern Africa, in order to ground some of the previous discussions in examples – a process which was very useful in developing an understanding of how the approach could be implemented in practice.
The broad context for southern Africa is that there is a trend towards a shorter wet season in many areas, and that there is a strong link between ENSO cycles and climatic variability. Maize was the crop looked at as it has a very important role in the region, and 2 different precipitation thresholds for planting and one for the end of the rainy season were identified:
- 25mm in 10 days (Early planting)
- 45mm in 4 days (Later planting)
Using these climatic indices allows an analysis of historical rainfall data to show any trend in the mean planting date and the mean date of the end of the rainy season, allowing trend in the length of the growing season to be examined. Analysis shows that in some areas of southern Africa the length of the growing season has decreased by as much as 30 days in the last 30 years. Starting with an analysis of trends in the growing season in different areas allows you to then narrow the analysis to areas which are close to the threshold length needed to grow their crop, and where the length of growing season shows a negative trend. These are the areas which are most vulnerable to further changes to the length of the growing season. It must be noted that the length of dry spells at different points in the season must also be looked at; for example if early planting occurs and there is then a subsequent prolonged dry spell (in effect a ‘false start’ to the rainy season), then the planted crops will fail.
Livingstone, Zambia
An earlier start and end to the rainy season was strongly correlated with El Niño years, but further analysis showed that early planting was often followed by a dry spell, and that there was no change in the dates when planting without a false start could occur. This means that in effect El Niño years reduce the length of the growing season below the threshold required to grow maize. Knowing this, and because El Niño events are predicted accurately in advance, it becomes possible to adopt different strategies during El Niño years, such as growing different cultivars, or using water storage methods to overcome early season dry spells. This is a basic analysis, as crops respond to soil moisture rather than overall levels of precipitation; however a more complex analysis combining precipitation, temperature and soil moisture indices could equally be undertaken. Issues to think about are how to incorporate these climatic analyses with other stresses leading to vulnerability, and the issue of the quality and availability of climate data. As a simple example of this, there may be strong cultural attachments to certain crops which mean that other crops which may require a shorter growing season are not actually suitable replacements. Other oscillations in the climate system, such as the Antarctic oscillation, also show correlations with rainfall over southern Africa. Mark noted that the rainfall signal from climate change is not expected to occur until much later than changes in temperature, so it is important to look not only at projected changes, but also when they are expected to occur.
Question and Answer Session with Mark Tadross
Q. The difference between statistical and empirical downscaling
A. Statistical downscaling
y= ax + b
y = rainfall x= atmospheric pressure
Empirical downscaling observes more variables and systems and therefore there is more room for error using this method.
Q. Data needed for downscaling
A. Daily mean temperature. {Daily (min and max) temperature. Daily precipitation (longitude and latitude)} 10 year record
Q. GCMs, model data and topography
A. Statistical downscaling will read topographical data well because it reads specific observations. The problem might be that there are fewer stations in highland areas but you can assume temperature change with height.
Q. Reanalysis data (CRU &NCEP)
A. CRU – observation on the ground and interpolated between them gives ideas of what really happens at the surface. NCEP uses model data (from atmospheric data compiled by balloons and satellite images) and forces the observed data (from the surface) to it so that it assumes normalcy as what is happening on the ground. NCEP is a GCM forced to look like the situation on the ground/in the real world. CRU and observe data would be useful in giving the statistical relationship between real and predicted events.
Q. CSAG and IPCC models
A. CSAG has chosen 11 of the 24 IPCC models and the basis of that choice has been that the chosen models are the only ones which have archived daily observed data, which is a requirement for the downscaling method used. There are 5 more models that will be added to the CSAG archive – More to add by Bruce Hewitson.
- Impact modelling using downscaled data sets
- Land races = non-genetically modified maize (this can be seen as an adaptation)
- WSI = Water Satisfaction Index
What parameters are important when dealing with missing data? Using other substitute methods such as using the large ecosystem approaches of Thornwaite.
Interpolation and data up scaling
- Uses GIS data to overlay crop yield and water satisfaction data to then be able to say which crops are suitable for growth in certain regions
- It is easier to get data per district/provincial level as opposed to national data. It is sometimes useful to collect the provincial and try to average what is available to it for the whole country
- The choice of methods of analysis – downscaled GCM data and GCM interpolated data. Does it really make a difference in terms of output which model is used? Does one need to go through all these processes for practical use/application?
- Socio-economic and management factors – how can they best be integrated into climate science data output(s)?
- Document the pitfalls of modelling given situations where there are missing data > typical of most African countries
Q. Interpolation vs. downscaling GCM data
A. Take a GCM data rid and looking at the value of the centre of the grid box to another grid between the two grids is therefore interpolated data. There is no added value to these smaller grids/points.
Session 7: Impact Analysis and Reflections
Friday, 4 April
Mid-Workshop Feedback and Overview
Participants were asked to provide feedback on three questions regarding the workshop and structure:
- What will you work on during the week? And what resources do you need to be able to do this?
- ‘I am looking forward to the sessions on interpreting CCE output- because I need knowledge on both using the tool and interpreting its results for risk communication activities.â€
- ‘More interpretation and trend analysis will be helpful (as in the case study presented by Mark Tadross on Madagascar)â€
- ‘Comparative analysis of downscaling methods and tools and methods for trend analysisâ€. I need some basic material on RCMs and a bit of ‘technical backstopping’.
- ‘I would like to write a briefing on the key messages from baseline analysesâ€
- What did you learn this week that is most useful for your work in the ACCCA technical assistance teams?
- ‘The importance of background context for interpreting climate change information.â€
- ‘What NCEP re-analysis actually is.â€
- ‘How you might match climate oscillations such as ENSO to crop seasons and important periods of growth.â€
- ‘Uncertainty issues:
- Be cautious with both observations and model: they both might be deceptive
- Look at climate change as a development issue, instead of a scientific problem.
- New analysis techniques/methods to study climate change.â€
- ‘Some of the science has been confusing. But highlighting the possible variables and parameters that we might need to been great. The activity on Tuesday afternoon on African case studies was useful and it would be useful to continue with this, and start to explore possible adaptation options based on that.â€
- What questions or difficulties remain in your mind after the first week?
- ‘I feel that we haven’t had much time to work on risk communications. However, in the sessions that we have done, it has been useful to draw links between these and how they may incorporate into the communications sessions.â€
- ‘Things are not as easy as I expected. I am now more realistic about climate information, for climate change adaptation.â€
- ‘I am bit confused about the usefulness of GCMs for climate change adaptation.â€
- ‘To what extent can I have confidence in downscaled data due to local effects?â€
- ‘How can we integrate the timescales? ie. Adapting to current variability but with long term trends in mind? Why are some models IPCC and others not?
Following this session, Sepo Hachingota of CSAG, presented some of his initial findings around the use of impact modelling using downscaled data. Key points from his presentation were:
- There is a real issue of missing data, and the availability of data which is appropriate to put into crop and hydrological models.
- Crop data is generally at a regional level, whereas downscaled climate data is point specific, so you must assume that the station is representative of the broader region, and that the average regional yield is representative of yield at the station.
- To what extent do non-climatic factors, such as price of fertilizer or political instability, influence yield?
- The data you are using must be appropriate for your purpose. For example if you need to know about changes in water availability at a watershed scale, then GCM data may be appropriate. If your concern is sub-regional changes in water availability for crops then data downscaled to the station level is more appropriate.
Sepo uses interpolation to scale up the results of crop models run at a station scale to regional averages. This raised a discussion about the differences between downscaling and interpolation:
Interpolation was explained as being an averaging technique, and can be used either to average up data from a point to a broader scale, or from a broad scale (for example a GCM grid cell) to a finer scale. Essentially, interpolation looks at an area in a grid cell and plots it’s distance to the centre of that cell, and to the centre of other neighbouring cells. It then uses this information to produce a value for that area which is an average of the values of the surrounding cells, weighted by their relative proximity.
Downscaling on the other hand is not an average, but uses the relationship between events at the broad scale (synoptic atmospheric processes) and conditions at the local level to infer local conditions from the broader processes.
Session 8: Interpretation
Monday 7th April
Fernanda provided a set of questions to answer when developing the baseline context of vulnerability and climate for a region, and a list of useful resources to use when answering those questions, including both websites and baseline datasets in the AWhere GIS software. The morning was spent working through some of these questions to develop the context for the case studies started earlier in the week, with the aim of presenting these to the group on Thursday. Fernanda also showed different members of the group how to use AWhere to produce maps of information such as crop yield, population density and average precipitation.
In the afternoon sessions Mark was shown a series of slides showing different graphs or maps of climate information or climate projections, and asked to interpret these. The slides all deliberately showed badly constructed figures where it was hard to fully interpret what information was being shown, but were all taken from published material. Key points taken from this session were:
- Figures must always state the units of what they are measuring and information about the time period they are showing.
- Figures must always name the climate projection, or average of climate projections that were used in the analysis
- It is important to show change rather than absolute values, as this makes it easier to compare the possible future climate with the present.
- Presentation is important and should be intuitive. For example an increase in red colour is associated with negative effects, so shouldn’t be used to show an increase in the growing season.
- If the variables shown are complex, an explanation of what they mean should be given.
Tuesday, 8 April
Bruce Hewitson led a discussion on some of the meta-concepts that everybody working on climate change needs to understand. This is a work in development and the concepts are open to discussion, however it was useful to discuss these, and working definitions for the terms as explored in the workshop are provided below.
Meta-concepts
- Uncertainty: structure of unpredictability of a system which can arise from a lot of resources, such as lack of knowledge, limit of understanding of the climate system
- Threshold: threshold is important for both physical and social-economical systems; it’s a transition between different states; tipping point in social sciences
- Sustainable development: meet the present development without compromising the future ability to develop
- Scenario: an action or event that might happen in future. there’re scenarios for emission, climate change, economy
- Scales: either in spatial of in temporal. climate change is about scales. it’s also related to uncertainty. for different community different scale matters.
- Risk: an assessment of lose and gain; based on multiple perspective, definition and usage; can not be quantified.
- Forecast and projection: forecast is to predict the state of the atmosphere for a near future, like next couple of days, next few months; projection is to predict the distant future that has larger range of possibilities and uncertainties; they are all about possibility and the reasons/evidences behind it.
- Multiple stressors: many constraining interactive factors that affect outcomes, and climate is one of them. The problem is, what are they? How do they interact with each other?
- Model: reduced complexity numerical system that simulates basic behaviour of climate instead of reality. It is getting more complicated, but not necessary better.
- Model sensitivity: magnitude of a response for a given forcing. as in global temperature equilibrium response to double CO2.
- Mitigation: actions of aiming at reducing impacts of climate change; to prevent dangerous climate change, reducing the magnitude of a damage impact.
- Signal to noise ratio: ratio between a signal, such as temperature change, to other source of variance. In climate change science, noise usually refers to natural variability.
- Feedback: process that response to a forcing that can either amplify or weaken the initial change.
- Ensemble: method that explorers the nature range of a system to study the uncertainty and possibility. There are multi-model ensemble, and multi-simulation ensemble.
- Envelope: along the time line into the future, there’s range of possibilities. related concepts: probability, variability, average, range, limit, distribution tails
- Downscale: scale transfer activity; the purpose is to relate large scale processes from GCM to regional/local climate response. There are two kinds of downscaling: dynamical and empirical, both of which depend on GCMs. dynamical downscaling is using RCM and powerful in studying the feedback processes; statistical is more accurate at stational scale and can capture first order responses better than RCMs. related concept: pattern scale, stationary issue, deterministic/stochastic variances
- Dangerous climate change: it is context specific and region specific, according to the consideration of degree of causing damages within a given context.
- Communication: exchanges of information and understanding in and between communities.
- Attribute: trying to put a cause to the change; or ascribing a cause to a defined feature, such as CO2 to 20th century temperature increase.
- Agency: people’s ability to act or express their own concerns and need and effect change; multiple scales from individual to community.
Session 9: The Climate Change Explorer and Interpretation
In the afternoon the Climate Change Explorer tool was introduced, with a focus on how to interpret the output of the tool rather than the basics of how to use it. Key to the session was to understand that the variables to look at will vary depending on the context of your project and the questions that you are trying to answer and that one must be able to interpret what different output from the tool shows. In addition to this several important points were made:
- The Future A period is 2046-2065 and the Future B period is 2081-2100. It was noted that these names are not intuitive and should be changed.
- All of the model output is for the A2 socio-economic scenario. This is due to practical reasons as this the scenario which most GCMs have run.
- Start from looking at the NCEP reanalysis curve as this is the best representation of current climate. If the control runs of a model do not broadly capture the shape of the NCEP curve then they should probably not be used. The magnitude of what they show is less important.
- The model which simulates the current climate most closely IS NOT NECESSARILY MORE ACCURATE IN IT’S FUTURE PROJECTIONS
- It is best to look at anomalies rather than changes in absolute magnitude, unless you are looking to see if an important threshold has been crossed.
- Where the models agree on the sign of change in a variable (e.g. increased precipitation) there is more confidence in that change.
- It is important to look at the right variables for your situation, and periods in the year where change would be most important. This is why it is vital to first develop the context for an area, so you can target the use of the tool.
Session 10: Uncertainty
Wednesday 9th April
Wednesday’s sessions were led by Bruce Hewitson, who explained the concepts around uncertainty in climate modelling.
Bruce started with the idea that perception of uncertainty can be important as well as the uncertainty itself. The example used was that if people are told there is a 1 in 10 chance of being run over by a bus when they leave the building many will opt to stay inside (this worked in our group!) but that a 1 in 10 chance of recurrent severe drought in the Cape Peninsula in 2030 is treated as a small risk. Uncertainty can be over the chance of a single event (for example crossing a threshold), recurrent events (the return period of a flood for example), discrete events (hurricane frequency) and complex events (for example the interplay of different factors that lead to drought).
It is extremely important to understand the range of uncertainty that exists, rather than just relying on one outcome chosen from many possibilities. It was also mentioned that some areas of uncertainty are likely decrease, but that some may not, for example the range of change in temperature for 2050 has changed very little since initial calculations were made over 20 years ago, so it is important to recognise that we need to work with this uncertainty. Some aspects of the climate system may be too chaotic to say with certainty where within a range of possibilities the system may end.
Bruce explained that models use different parameters to simulate some aspects of the climate system, rather than underlying physics. These prescribed variables are essentially educated guesses, which introduce uncertainty into the models. This is why experiments such as climateprediction.net are using many thousands of model runs and changing the values of the parameters slightly to produce a full range of possible plausible changes. There is also uncertainty relating to the initial conditions from which the models run, as changing these can change model output. This is where the idea of running the same model multiple time with different initial conditions comes from (multi-ensemble model runs) – to give the range of projections from each model. The more simulations there are, the more confidence there should be that we are looking at the full range of uncertainty in the system, although it may be that more models are needed in order to capture the full envelope of uncertainty.
The different socio-economic scenarios can introduce uncertainty, however they diverge very little until 2050 so medium-term uncertainty is low. In addition, many models now simulate what happens to climate under a certain concentration of carbon dioxide (for example 450ppm) rather than trying to guess how emissions will change.
Very little difference has been found between the 2 main techniques used for empirical downscaling, those of Rob Wilby and Bruce Hewitson. There can be large differences, however, between these techniques and the dynamical downscaling performed by RCMs. In a similar way to GCMs, different RCMs can give very different results, so may not help to reduce uncertainty in climate projections. If using impact models (crop models for example), these may introduce additional uncertainty into the projections of change. The key question to think of is how relevant is the uncertainty to your context; in many cases if there is confidence between the models about the direction of change, this will be enough to make a decision; uncertainty doesn’t mean that we can’t do anything.
There is a 3 step process to understand a large amount of the change which will occur at your site:
- Understand the historical climate and any trends shown
- Look at the multi-model GCM results (such as those shown in the IPCC reports) to understand the regional level
- Look at downscaled data and see if it makes sense; is it consistent with the larger scale processes?
One way of looking at uncertainty is to take an envelope analysis. This involves taking multiple runs (ensembles) with different conditions, of multiple models, and looking at the distribution of the results. There will be some agreement about the expected changes, for example all the models might say it will get warmer in a certain region, so there is high confidence in this change. The models will also disagree about some changes, for example in precipitation, but by looking at distribution you can see how many project each type of change. The idea of envelope analysis however is not that just because there are lots of runs which give a specific type of result (for example warmer and wetter) this is the most likely result. Rather it is based on the fact that we can’t tell whether one run is better than another, so instead you take the complete envelope of possibilities shown by the model runs, and understand that the future climate will lie within this range of changes. An example of the value of not ignoring results which appear to be outliers comes from the IPCC 4th assessment report. Only 1 model in the IPCC simulates 20th century climate in the Sahel accurately – it is an outlier compared to the rest, but is actually the most accurate. Finally it was mentioned that the UK climate impacts programme (UKCIP) in it’s next report is planning to put probabilities on future changes. This was discussed and the fact that there are lots of assumptions underlying the calculation of these probabilities, coupled with the fact that even if these assumptions are correct the detailed data needed for these calculations are not available for the rest of the world , mean that assigning probabilities is not possible for most areas.
Bruce then went on to talk about downscaling, both an overview of how to do empirical downscaling, and a comparison of empirical and dynamical downscaling. Much of this was discussed on the Thursday of the 1st week with Mark Tadross, but it was useful to hear another explanation of it.
It was emphasised that it is important to relate results back to the large-scale processes which drive the climate system and to think about the interlinked nature of the system. The changes given by downscaling must always be explicable in relation to the larger process; is there a physical mechanism which could drive this change? In areas where different models show differences in the sign of the expected changes it is important to build resilience of the system and capacity to adapt rather than implementing an adaptation measure which is dependent on one direction of change. Feedbacks will be most important in areas where projected change is small, as they could change the sign of the change (for example from increased to decreased rainfall). If feedbacks are identified as important in such areas it is possible that work following on from ACCCA projects might want to use RCMs to look at what effect the feedbacks would have. In most cases, however, feedbacks will not greatly affect the changes driven by larger processes.
Session 11: Communication for Disaster Mitigation
Thursday, 10 April
In the morning Leigh from the Disaster Mitigation for Sustainable Livelihoods program at UCT gave a talk to the workshop about risk and vulnerability, and how to communicate and increase awareness of risk. Some key points from the talk were that trust is a vital part of the process so partnerships should be made with trusted local organisations, risks from climate change should be framed starting from the concerns of the community and simple communication methods are often the most effective. Leigh talked of the difficulty of getting true community participation because certain groups may not be able to dedicate the time needed for the participatory process, particularly if they must work. In this case follow up work may be needed, or if it is impossible to get true representation then the team must be aware of the bias of their sample. She also noted that the facilitator should be appropriate for the situation, for example women will be less likely to share their concerns if men are present.
(0) Comments
There is no content