Developing regional climate change messages using multiple lines of evidence
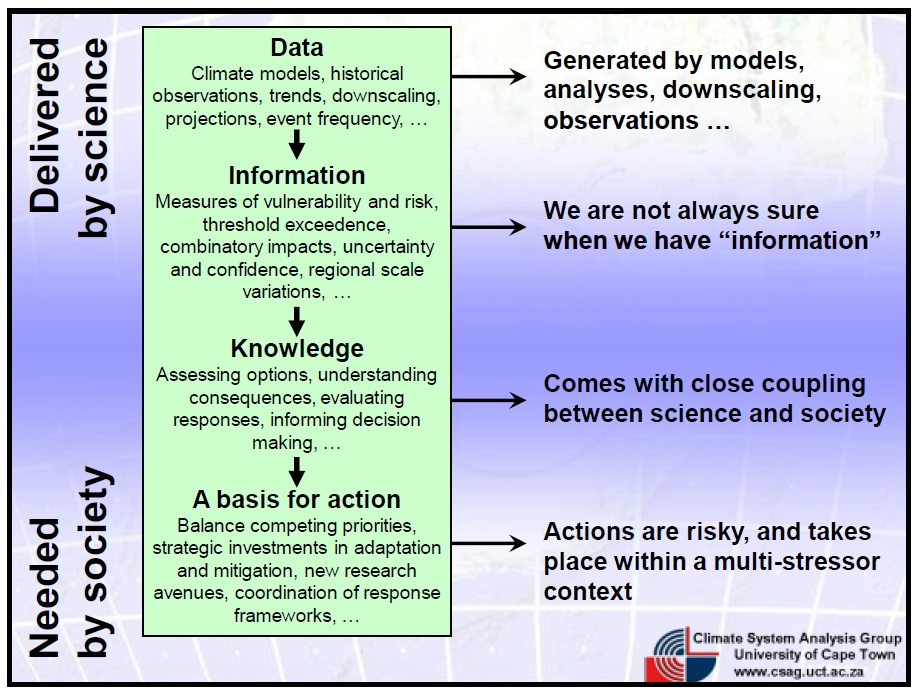
To build a message about changes in the regional climate (rather than the global climate) the typical questions you will need to answer are:
a) What is the typical seasonal climate of temperature and rainfall? b) What is the natural variability? c) How do I know this? d) How has climate changed in the recent past? e) What are the driving large-scale processes for this locations climate? f) What local forcing factors are relevant to the regional climate? g) Are there important tele-connection factors to consider? h) What is the projected future climate change for this location, by what method are these projections made? i) Are there contradictions between information about past changes, future changes, and the various sources of this information? j) How will I assess the quality / reliability of my information? k) What are the drivers of vulnerability in the region to such change in the climate? l) Is it possible to characterize the probability distribution of key climate variables to see changes in likelihood of various outcomes?
In order to answer the above questions and develop a set of robust and scientifically defensible messages about the climate changes in the region it is important to draw on information from 4 sources:
1. Coupled Atmosphere-Ocean Global Circulation Models (GCMs) e.g. CMIP3 data archive and soon CMIP5; 2. Downscaled GCM model results, either using Regional Climate Models (RCMs) and/or Statistical Downscaling; 3. Knowledge of the physical processes governing regional (and local) responses to global changes in the climate system; 4. Statistical analyses of observational data records that reveal any recent historical changes in the climate as measured on the ground.
Each source has different attributes of signal and noise, limitations on interpretation and degrees of uncertainty. For example, GCMs are good for looking a large-scale patterns and feedbacks in the global climate system but are too coarse in their resolution to say anything useful about the specifics of what is happening or likely to be experienced on the ground in any given place. GCM grid cells do not represent the climate at any given point within that spatial extent of that grid cell, rather the grid cell values are an area average. Given this, it is NOT valid to interpret a GCM grid cell at a point! Point location variation is not represented by GCM grid cell data. Downscaling adds local specificity to the GCM results, generating results for point locations or for grid cells covering a much smaller area (i.e. higher resolution), depending on the downscaling method used.
However, when looking at downscaled information it’s easy to lose sight of the bigger picture in terms of spatial variability and explanatory power for what is driving the expected changes. Also, downscaled information is not available for all regions, especially not in places where there are minimal observational data records (source 4). As with most things, climate science is not equally distributed. There are differing amounts of information from these 4 sources available for each region. Many of these data and knowledge sets remain scarce over large parts of Africa. However, there are large international collaborations currently underway to redress some of these inequities (for example the CORDEX programme).
Bringing together information from all 4 sources makes it possible to develop a fuller and more robust picture of the climatic changes occurring in a given region and tackle questions of what the impacts of such changes are and might be into the future and what to do accordingly – what regulations and policies to put in place, what investments to make and what actions to take in terms reducing greenhouse gases (mitigation) and preparing to accommodate the changes that do occur to minimize the impacts (adaptation).
When it comes to understanding how climate conditions relate to the living and working realities of people and promoting various climate adaptation and mitigation measures, it may well be important to relate the above-mentioned scientific sources of climate information to the climate observations, perceptions and narratives of those who experience the climate first-hand, who have additional information about impact pathways and other reasons for why things are done in a certain way.
Summary of the approach being suggested
A: Characterize baseline observational climate as best as possible – use station data, gridded data, derivatives
B: Characterize process change to inform understanding – consider circulation change as a means to gain confidence in location- specific climate
C: Use as many models as possible – ideally focus on model simulations run under common forcing
D: Downscale where possible – RCM downscaling and/or statistical downscaling; different strengths and weaknesses
E: Clearly understand the limits to available data
F: Consider how the available science relates to people’s experiences and perception of the climate and how it impacts them
Credit
This article was developed from a module presented at the 2012 Winter School by Professor Bruce Hewitson, head of the Climate Systems Analysis Group (CSAG) at the University of Cape Town.
(0) Comments
There is no content