Learning within & about climate science: What has transdisciplinary engagement through FRACTAL taught us?
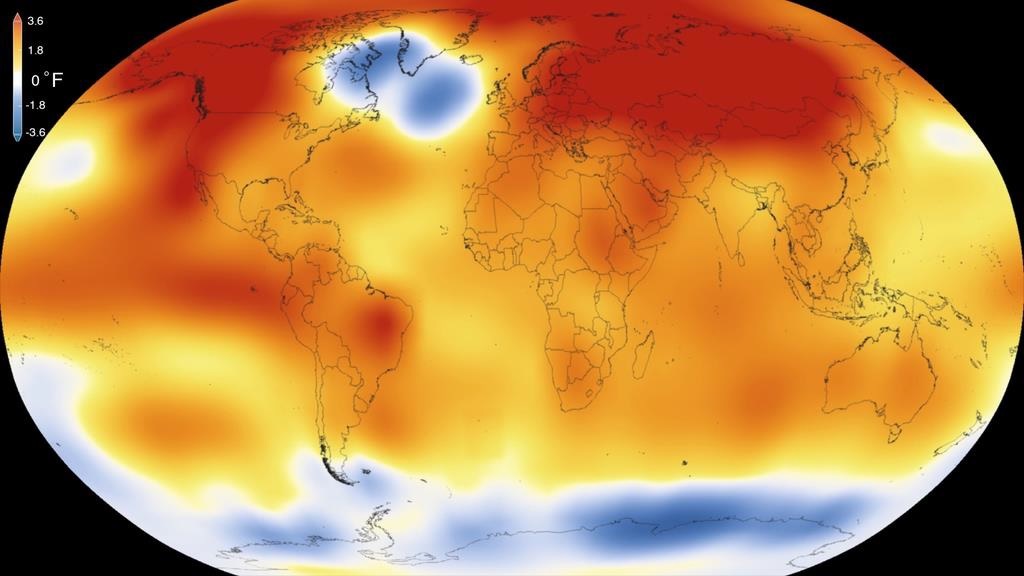
Introduction
How does what we believe shape what we know? What power do we wield knowing the things we know as climate scientists? What are the consequences of our beliefs, framings, assumptions and values? And what do these all mean for FRACTAL?
It’s useful to interrogate where and when learning happens in these activities, and that there are many ways to learn. We should consider this when trying to understand learning.
This briefing note* provides key discussion points from the second Future Resilience for African Cities and Lands (FRACTAL) learning webinar, during which the team reflected on the role of learning in integrating climate science into decision making. Access the podcast here. This article discusses transdisciplinary engagement;read this guide on how to establish transdisciplinary learning processes and co-production of knowledge. Finally, you can access an article highlighting the FRACTAL principles of transdisciplinarity, co-production and co-exploration here.
*Download the full document from the right hand column. The key messages from the document are provided below. See the full text for much more detail.
Learning processes in climate sciences
This FRACTAL learning webinar started off with a introduction on learning processes from Chris Jack. This introduction is summarised below.
Learning processes
The dictionary definition of learning is the “acquisition of knowledge and skills through study, experience or being taught”. But what does it mean to learn in the climate sciences? Examples of simplifed learning processes in the climate sciences include:
- learning something one didn’t know previously (e.g. to use a provocative statement, that some parts of Africa might be cooling);
- adding evidence to ones existing belief (e.g. I now have evidence of increasing rainfall in Cape Town);
- adding evidence to contradict ones belief, which leads to new beliefs; and/or
- being able to do something better through skills development or experience.
Not all climate science activities entail learning (based on the processes described above). For example, running a climate model: the output or experience might result in learning but the model on its own is not a case of learning. Similarly, presenting projections to a decision maker may or may not involve learning on the part of the climate scientist as well as the decision maker.
It is useful to interrogate where and when learning happens in these activities, and to appreciate that there are many ways to learn.
The continuum of science
There has been a lot discussion about what counts as evidence when so much subjectivity is introduced into the learning processes. These discussions are aligned with academic discourses about the continuum of normal to postnormal approaches to science and enquiry.
- ‘Normal’ science is characterised by principles, values, sets of assumptions and framings on which many people agree. This type of enquiry is suitable in instances when uncertainty and decision stakes are low.
- The next on the spectrum is ‘consultancy science’, during which uncertainty is incorporated into decision making.
- Last on the spectrum of approaches is ‘post-normal science’, which is appropriate when complexity is irreducible. This is much of what FRACTAL is dealing with.
Ethical epistemic challenges for climate science
How does what we believe shape what we know? What power do we wield knowing the things we know as climate scientists? What are the consequences of our beliefs, framings, assumptions and values?
These are important ethical epistemic challenges for climate science. As an example of this complexity, the decision scaling work in FRACTAL is pointing to the fact that climate change won’t be a big risk for flow coming out of the Kafue River, which supplies water to Lusaka. Attempting to integrate this message into the discussion in Lusaka, which involves lots of concern related to the flows of the Kafue in the future, has been an interesting and challenging process. Although climate might not be a major stressor according to the modeling work (though more work needs to be done), the way in which climate will intersect with development in the future, and possible many other stressors, is unknown… So, the question is, how strongly do we push the science output into a space that’s a lot more complicated?
Lessons learned through FRACTAL
After an introduction (see above), the team broke into two groups (climate scientists and nonclimate scientists) to discuss what team members have learned about climate science through FRACTAL. The key lessons for learning outside the climate science discipline are that:
- many cities are incredibly vulnerable to the effects of natural climate variability, before we even consider climate change;
- there’s nothing like a good disaster to stimulate learning about how climate and society intersect;
- there are few entry points for climate information in current decision-making structures and processes;
- the narrative process has helped climate scientists learn a lot about things outside of their discipline;
- many potential users of climate information are not sure what they need to make resilient decisions;
- climate is not the main stressor in cities;
- language is a big challenge;
- using climate information in city decision making is challenging, and the learning labs are helping to facilitate discussions about this;
- there is a need to find a common point at which academics and practitioners talk to each other, setting the same goals;
- climate information can be very scientific and difficult to use;
- stakeholder engagement and ongoing interaction is very important to support translating science into everyday language;
- FRACTAL team members have felt very challenged through working in such a complex programme;
- the concept of receptivity is important.
Wrap-up reflections
Reflecting on all of the lesons learned (see above), the wrap-up discussion emphasised that:
- only when one understands the detail of a socio-economic system such as a city (or part of it) does one begin to understand the value of climate science, and where and when it is useful and usable; and
- these systems need to be explored in enough detail to know where climate science fits in.
As an example, the intersection of the recent drought in Cape Town and water management in the city; we can only begin understanding the impacts of the drought when we understand decisions related to water management. This begs the question; is there only one way of undertaking co-production of knowledge for climate resilience, at least in cities? (i.e. following these long, resourceintensive, emergent learning processes). As a FRACTAL team, it’s hard to imagine how we would do it otherwise.
This briefing note was produced by Alice McClure, from the Climate Systems Analysis Group, University of Cape Town, with inputs from Chris Jack (UCT) and many other FRACTAL team members.
Suggested citation
Future Resilience of African CiTies and Lands (2018) Learning within & about climate science. Future Resilience of African CiTies and Lands: Cape Town, South Africa.
Related Articles
Related resources
- FRACTAL project website
- FRACTAL: Future Resilience for African Cities and Lands
- Co-producing climate knowledge – Great in theory, but how about practice?
- Africa's Climate: Helping decision-makers make sense of climate information
- Transdisciplinarity, co-production, and co-exploration: integrating knowledge across science, policy and practice in FRACTAL
- Receptivity and judgement: expanding ways of knowing the climate to strengthen the resilience of cities
- Co-exploratory climate risk workshops: Experiences from urban Africa
- Designing Knowledge Coproduction for Climate and Development
- The Tandem framework: a holistic approach to co-designing climate services
- A guide to Effective Collaboration and Learning in Consortia
- FRACTAL Podcast Series - Exploring transdisciplinary approaches to support resilience and adaptation decision making
(0) Comments
There is no content