Robust Decision Making: XLRM framework
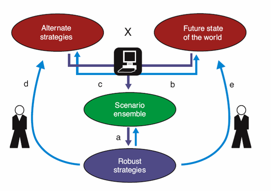
Robust Decision Making
RAND corporation has been developing a tool to carry out robust decision making as an example of computational multi-scenario simulation. Decision making based on the probabilistic approach mentioned above focuses on identifying uncertainties as accurate as possible. In contrast, decision making for uncertain future requires more holistic approach, i.e. robust decision making needs to consider as many as possible vulnerable factors and scenarios and identify the most acceptable vulnerable situation (Lempert, Nakicenovic et al. 2004). For example, a decision maker may sort all vulnerable scenarios into the ones his/her strategies and policy perform well and the other ones, which these strategies do not perform well. Then, the decision maker characterise the set of poorly performing scenarios and identify the futures, which their selected strategies and policies are vulnerable.
The robust decision making is still consistent with traditional optimum expected utility analysis, but the order is the other way around (Groves, Lempert 2007 p. 76). While conventional analysis characterise uncertainties to before ranking options, their decision making approach, the robust decision approach starts from selecting decision options and then estimate utilities of options to identify potential vulnerabilities of potential strategies. In other words, the robust decision making is different from conventional sensitivity analysis. The conventional approach studies the variability of outcomes against many input variables. Instead, their robust decision making is to find strategies, which perform well insensitively to the most significant uncertainties. Its procedure is explained detail in Lempert et al. (2006), so I put short summary of each step below:
- Identify initial candidate robust strategies: decision makers proposal initial strategies and ranked them by the approach mentioned above although the initial ranking may change as these strategies are examined with different probability weightings.
- Identify vulnerabilities: the clusters of future states, which candidate strategies perform poorly, are identified by statistical or sensitivity analysis, i.e. finding futures where strategies exceed satisfactory levels by using patient rule induction method (PRIM) (Friedman, Fisher 1999).
- Suggest hedges against vulnerabilities: alternative strategies are ranked again based on the performance of each future cluster. The ranking forms vulnerability frontiers.
- Characterize deep uncertainties and trade-offs among strategies: as the strategies in the trade-off curve is dependent on the vulnerable future states. Therefore, this information will be utilised to characterise deep uncertainty and to choose new candidate strategies.
- Consider improved hedging options and surprises: repeat these steps and consider characteristics of surprises.
The XLRM Framework
The theoretical framework for research is based on the concept of Robust Decision Making (RDM), whereby robustness is described as a management decision strategy made against multiple future scenarios and critical uncertainties in order to enhance the flexibility and likelihood of a successful outcome. This approach is favored as an alternative to traditional utility optimizing, probability-based decision-making given circumstances of deep uncertainty confronting program design features and exogenous variables affecting the sustainability of Oxfam’s microinsurance scheme. It is important to stress that the RDM model work is meant to be part of an ongoing process of decision-making that informs strategies that are ultimately chosen by decision-makers in the context of other, often non-quantitative, factors for consideration, e.g. equity, politics. The RDM approach we’ve adopted from RAND is based in what is called an XLRM framework whose components are defined as follows (Lempert et. al. 2003):
- Exogenous uncertainties (Xs) are factors outside the control of decision-makers that may nonetheless prove important in determining the success of their strategies.
- Policy levers (Ls) are near-term actions that, in various combinations, comprise the alternative strategies decision-makers want to explore.
- Relationships (Rs) are potential ways in which the future, and in particular those attributes addressed by the measures, evolve over time based on the decision-maker’s choices of levers and the manifestation of the uncertainties. A particular choice of Rs and Xs represents the future state of the world.
- Measures (Ms) are the performance standards that decision-makers and other interested communities would use to rank the desirability of various scenarios.
The below figure demonstrates the iterative nature of an RDM process based on XLRM (see chapters 3-5 of the RAND Corporation’s publication, ‘Shaping the next 100 years’ for further description of RDM, link below):
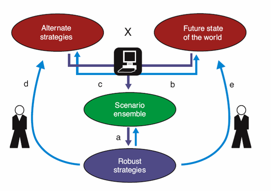
References
- FRIEDMAN, J.H. and FISHER, N.I., 1999. Bump hunting in high-dimensional data. Statistics and Computing, 9(2), pp. 123-143.
- GROVES, D.G. and LEMPERT, R.J., 2007. A new analytic method for finding policy-relevant scenarios. Global Environmental Change, 17(1), pp. 73-85.
- LEMPERT, R., NAKICENOVIC, N., SAREWITZ, D. and SCHLESINGER, M., 2004. Characterizing Climate-Change Uncertainties for Decision-Makers. An Editorial Essay. Climatic Change, 65(1), pp. 1-9.
- LEMPERT, R.J., GROVES, D.G., POPPER, S.W. and BANKES, S.C., 2006. A General, Analytic Method for Generating Robust Strategies and Narrative Scenarios. Management Science, 52(4), pp. 514-528.
- LEMPERT, R.J., POPPER, S.W. and BANKES, S.C., 2003. Shaping the Next One Hundred Years: New Methods for Quantitative, Long-Term Policy Analysis. RAND Corporation.
(0) Comments
There is no content